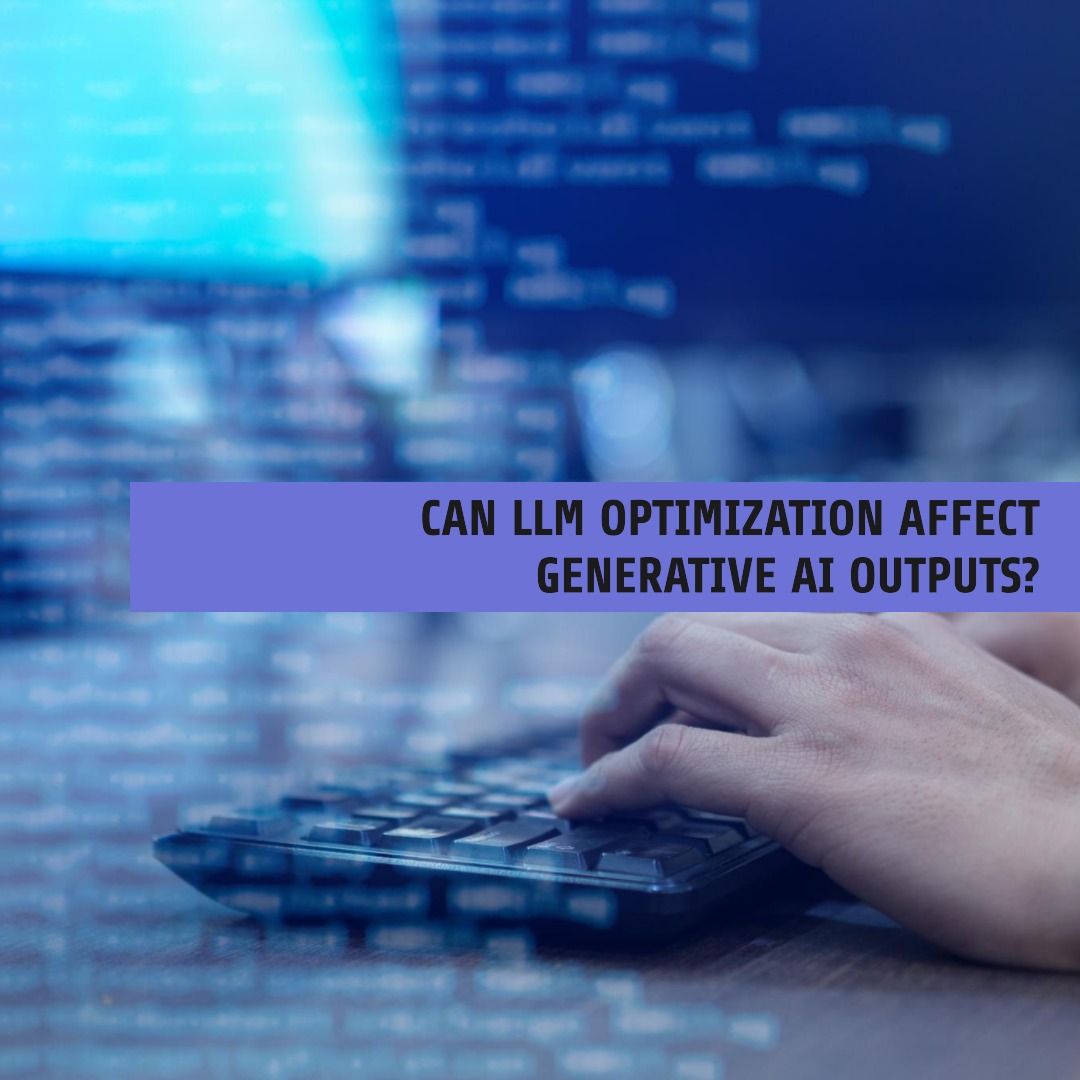
Can LlM Optimization Affect Generative Ai Outputs?
Machines can now write code, produce art, compose music, and create content due to the amazing advancements in generative AI in recent years. Large datasets have been used to train these AI systems, including GPT-3 and its offspring, which can produce language that is remarkably accurate and human-like. The possibility of unwanted or skewed results, however, remains a persistent worry. Do you have the ability to affect generative AI so that the results meet your goals? In the current discussion over-optimizing the Large Language Model (LLM), this subject is essential.
Learning to generate text is accomplished using LLMs, such as GPT-3, which are neural network-based models that anticipate the subsequent word in a sequence depending on the previous context. Since these models are trained on massive internet text datasets, they may generate language that reflects the prejudices and stereotypes found online due to the diversity of the training data. In generative AI, the question of "influence" has many facets. Users want to be in charge of the results, but AI engineers also need to balance user involvement with ethical problems.
It is possible to modify the results of generative AI in a number of ways:
One of the most popular methods for influencing AI is through prompt engineering, which involves creating a customized question. Your input can direct the AI toward the intended results by using word choice, structure, and context. For instance, you can format your prompt to have the AI provide an impartial synopsis of a subject.
Temperature and MaxTokens: The majority of LLMs have settings for things like "temperature" and "max tokens." One can control the output's randomness with the temperature parameter. more values lead to more unpredictability, whilst decreasing values produce a more predictable outcome. To limit how long the generated text is, you can set the max tokens. You may customize how the AI responds by changing these parameters.
Using rule-based systems to filter or sanitize AI outputs is a technique used in some applications. To stop the AI from producing damaging, prejudiced, or improper content, these guidelines can be programmed. Though frequently criticized for possibly stifling creativity, this strategy works well.
Enhancement: Using customized datasets, developers can refine already-trained models to get them closer to meeting particular needs. More control over the AI's behaviour can be achieved by fine-tuning, but it calls for a significant amount of extra information and knowledge.
Acquiring total control is not always feasible, even if these methods can influence AI outputs to some degree. Even with generative AI, unexpected or biased material can still be produced. Its training data's inherent biases restrict the amount of impact that can be applied to these models.
Maintaining an ethical balance while accounting for user impact can be difficult. Abnormal uses of AI, such as the creation of damaging material or the dissemination of misinformation, may be made possible by excessive user influence. To reduce these hazards, ethical AI research is being pursued. Aiming to limit undesirable uses, clarify restrictions, and lessen biases in AI models, developers are working hard.
These issues have been actively being worked on by OpenAI, the company that created GPT-3. To lessen overt as well as covert biases in their models, they have taken action. OpenAI has undertaken research investments to guarantee that its AI systems uphold the values of its users. It has also made an attempt to solicit public feedback on the use of AI in particular situations.
Users' accountability plays a significant role in shaping AI outcomes. The potential and constraints of generative AI must be understood by users. When it comes to taking AI-generated content at face value, they should be wary of accepting it at face value and critically assess the outputs, questioning their sources.
To sum up, LLM optimization seeks to reconcile ethical concerns with user influence. Complete control over generative AI outputs is still challenging, despite methods like quick engineering and parameter tweaks being able to direct outcomes. On the one hand, users should use AI-generated content with caution and responsibility, and developers should keep working to reduce biases. One day, generative AI will be a useful tool that helps us and entertains us without causing harm or spreading false information. Therefore, its development and responsible use will be essential to ensuring that this happens.